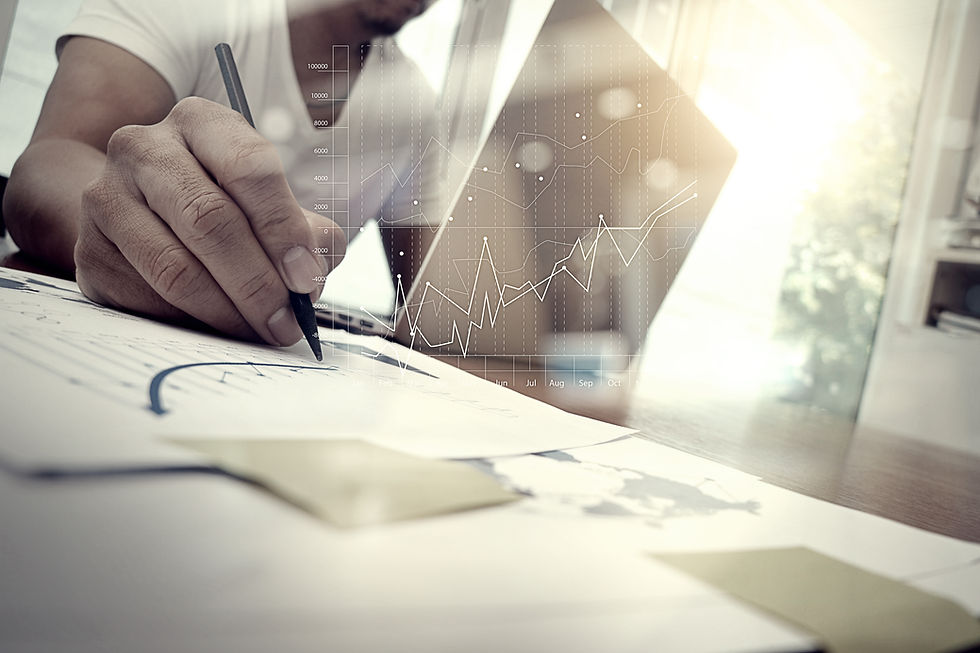
Integrity digs are performed to take actions to repair the defects identified through Inline Inspections. These actions are necessary to repair the sections of the pipeline that are likely going to fail and cause incidents. These digs are not pre-scheduled and integrity specialists have to plan them every year.
But there is no straight and effective method to schedule these digs and prioritize repairs in the most time and cost-effective manner. This results in either doing too many digs or not digging the necessary sections. Both of these scenarios have consequences.
Performing digs that are not necessary and that could be deferred negatively impacts company bottom lines and operations. Not digging the problematic sections early enough will result in pipeline failure, and all the environmental and financial consequences that come with it.
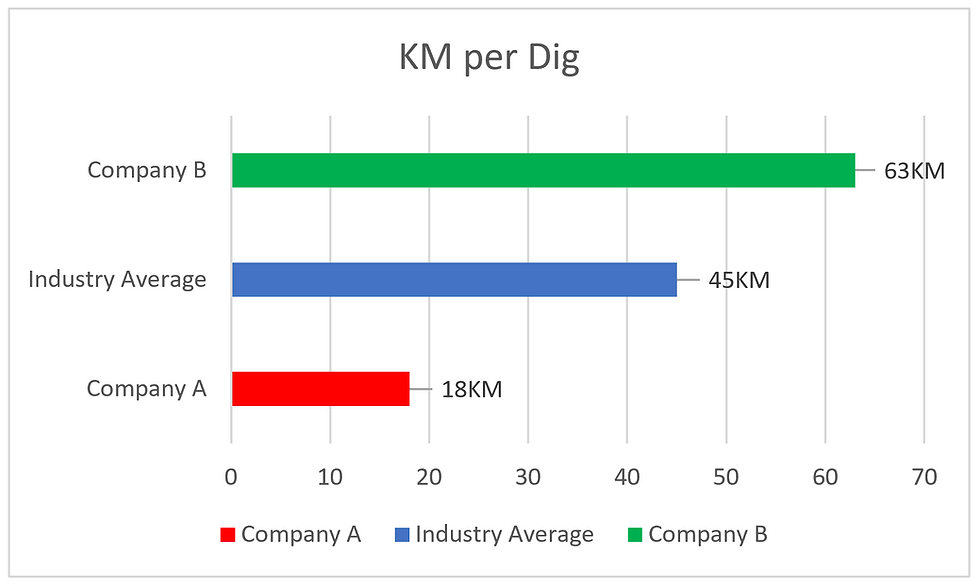
The graph compares the number of digs performed in 2018 by two pipeline operator companies and the industry average from CEPA.
Why did ‘Company A’ perform digs every 18KM while the industry average is one dig per 45KM? How many of these digs were unnecessary or could have been deferred?
Integra leverages its subject matter expertise in pipeline integrity to identify the roadblocks to effective integrity dig planning, and has addressed them through advanced data and analytic methodologies.
Generic ILI Tool Error
The main resource used to make the decision to repair a defect and perform a dig is the data collected through Inline Inspections (ILI).
ILI measurements of detected anomalies do not always represent the actual size of that anomaly and are vulnerable to a certain degree of errors. Tool performance is not the same for different vendors and technologies, nor is it the same for pipelines based on their characteristics and operating conditions. Currently, the majority of the pipeline industry uses vendor-reported errors and industry default values. These values may be good starting points, but are not the most accurate measures for a time and cost-effective dig plan.
Integra leverages Machine Learning algorithms, using previous ILI and dig data to model tool errors for each tool type/technology for different pipelines.
Scattered Data
Other than ILI data, there are other data sources that integrity specialists consider when planning a dig, such as previous dig information, failure history, interactive features, etc. These data sources are in different formats and scattered across different places.
That makes the process of extracting required information highly time consuming and cumbersome.
Integra’s Digital Hub™ platform, with its cloud computing capabilities, makes it possible to bring all the necessary data sources for dig planning together, extract the required information, and generate actionable insights.
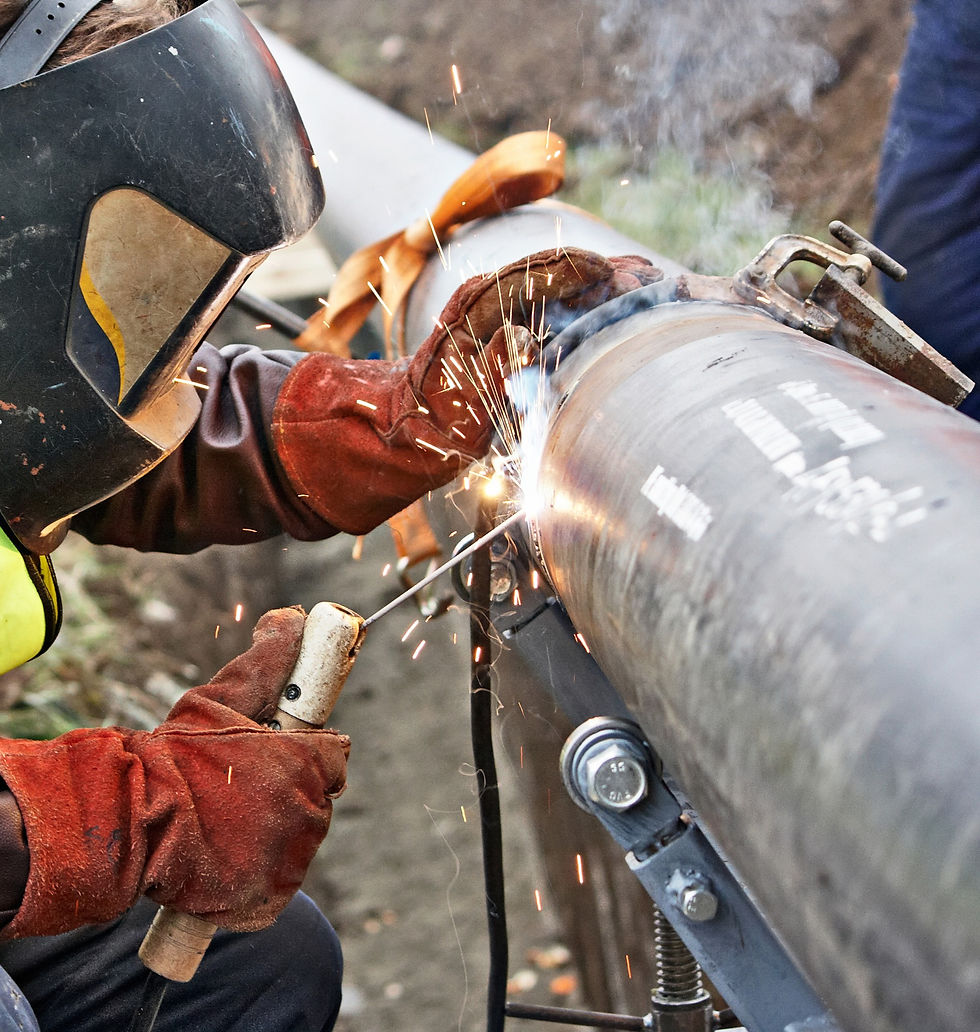
Manual Process
The process of generating a dig plan is highly manual and, again, with data being scattered, extracting the required information is painstakingly cumbersome.
Together, they increase the chance of human error in planning and prioritizing repairs. Compliance assessment of defects is one of the main elements of dig planning, requiring integrity specialists to follow certain industry best practices to determine the severity of the defects and prioritize repairs. This manual process greatly reduces the engineer's productive time.
Integra has developed Machine Learning algorithms to automate and semi-automate manual processes in dig planning that reduces the chance of error.
Ineffective Growth Rates
The growth rate of defects is one of the most important and, at the same time most complicated, factor in dig planning.
ILI runs are not done every year to monitor the growth of defects over time, and digs are not always conducted immediately after the ILI. In current calculations, growth rates are assumed to be linear and constant across the entire pipeline, which results in not accurately accounting for increases in the size of defects over time. This means inaccurately planning repairs. Growth rates are different for pipelines based on their pipe specifications, pipe environments, and operating conditions.
Integra has developed methodologies to reduce the uncertainties in growth models by implementing Machine Learning and Simulation techniques in growth rate calculation, using industry standard values and generating customized growth models when Run-to-Run analysis are not available.
Inaccurate Probability of Exceedance
Probability of Exceedance (POE) is the likelihood that a defect detected by ILI exceeds the acceptable criteria. POE is the main driver for a number of integrity decisions, such as dig planning and prioritizing. Current methodology to calculate POE is very generic and doesn’t take into account variations in pipe specifications and uncertainties in ILI measurements. Defects growth rates are also linear and constant across pipelines. These result in inaccurate POE and time to failure of defects for timely dig planning.
Integra takes all the errors and variations in parameters, such as ILI tool error and growth rates, into account and has developed an innovative solution to simulate millions of scenarios for POE and forecast the time to failure for each defect.
Comments